Simultaneous localization and mapping (SLAM) can be a powerful tool, delivering information for both robot navigation and operations management. Reliable visual SLAM can run on inexpensive hardware, making it relatively easy to deploy and scale.
In 2016, Andrew Davison, Stefan Leutenegger, Jacek Zienkiewicz and Owen Nicholson co-founded Slamcore while researching “spatial AI” machine vision for robots at Imperial College London.
Leveraging his experience of transforming early-stage technology startups into production systems, Nicholson said the team spent the next few years spinning out from the university, building their core technology and developing a product that can have a real impact on the world.
Now, Nicholson is the CEO of Slamcore. He said visual SLAM technology has been implemented in the consumer space for quite a while, in products including autonomous vacuums as well as augmented reality and virtual reality (AR and VR) headsets. However, he said visual SLAM has been slow to enter the industrial space because, “It’s harder in many ways.”
The challenges of creating vision-based SLAM for industrial applications
Nicholson said implementing visual SLAM in logistics warehouses and manufacturing facilities is challenged by the scale of industrial environments.
“Knowing where a vacuum cleaner is in a front room is difficult,” he said. “But knowing where an AMR is in a one million square foot warehouse is literally orders of magnitude more intense.”
Compared to measurement-based systems, like lasers or lidar, vision-based systems can be a computationally expensive way of providing information.
“And if you're not careful, the computation and the memory requirements to do that in real time can explode, especially at the scale of a factory or a warehouse,” Nicholson said.
“You can do it in a way where you take shortcuts on accuracy and reliability,” he added. “But at the end of the day, if these systems don't work and they're not bulletproof, then you haven't got a product.”
Another challenge is that warehouses might have inconsistent wireless network connections, or none at all. Nicholson said Slamcore decided to develop software for edge computing, with additional data management and sharing features enabled through the cloud.
On the edge, Slamcore’s software solves critical problems, such as determining if a robot is about to crash into something, or finding the quickest path between two points.
“What we're finding actually is the challenge - and this is partly why it is such a challenge - is because it has to run on the edge. You can't just cheat a bit and spin up another AWS core.”
The overall challenge Slamcore needed to address was how to deliver a workable visual SLAM for real-world industry beyond proof-of-concept. And, it had to perform on hardware at a reasonable cost.
Off-the-shelf hardware keeps costs low, deployments fast
Implementing vision-based navigation systems requires delivering both software and accompanying hardware. But building custom sensors can drive up the cost significantly.
“We've got a firm belief that the only way robotics are going to scale is if we use hardware which is readily available,” Nicholson said.
Slamcore turned to the consumer electronics market to source its cameras because the high production volume keeps hardware costs down. The company focused on making consumer-grade, off-the-shelf hardware industrially robust.
Nicholson said using off-the-shelf hardware was a commercial decision, not a technical one. Better hardware would enable Slamcore to build better visual SLAM software, but more affordable hardware makes sense for commercial applications.
“One of our things we've been very strict about is using affordable hardware,” Nicholson said.
Slamcore is developing two product lines:
- A software-only system
- And a hardware-provided one
While providing software alone got the spatial awareness product into robot users’ hands, Nicholson said that process requires calibration to get systems running.
Instead of installing and fine-tuning the software for legacy hardware, Slamcore can get new deployments up and running in a couple hours. “We did trials in the last month, where we had people on site and systems running by the end of the day - actually by the end of the first morning shift - which is kind of unheard of in this space,” Nicholson said.
In terms of technical specifications, Slamcore normally shoots video at 30 fps with relatively low-resolution cameras, such as those outputting 1024 by 768 through VGA. “You don't need anything more expensive than that, and that's what keeps costs down,” Nicholson said.
One feature that slightly raises costs is global shutters. Nicholson said rolling-shutter cameras make sense for consumer-level SLAM applications. But within the industrial space, the potential value both in operational cost savings and injury prevention justifies a few extra dollars for global shutters.
“This could ultimately improve your efficiency by 20% for the entire site, and it could reduce your number of accidents… by enough to really care about,” Nicholson said.
Vision-based localization can boost autonomous fleet scaling
Nicholson said some SLAM systems need a “teach and repeat” operational style, meaning they need to re-map when changes occur. “It's very hard to scale in that way.”
Slamcore’s hardware boxes can be mounted on existing vehicles that are already deployed. As the vehicles move around the facility, the system collects enough data to create an initial map to work off.
“Once that's done, it's done for life, and you don't have to do any remapping,” Nicholson said. “You can then bring online as many systems as you want, with just a single click to transfer the data from one box to another.”
Nicholson said positioning is the fundamental backbone of a spatial awareness system, for tracking both robots and manually-operated vehicles. “And actually, you need to do both.”
He added the most important assets for warehouse operators are manual forklifts, which move most of the value of goods.
“That's what's stopping us from scaling with a lot of robots,” Nicholson said. “You can't have robots just running around, doing their own thing and slowing down… manual forklifts.”
For example, if a robot’s localization fails and it gets lost - blocking a drive aisle or input and output bays - that could bring a customer’s entire production line to a standstill. “That trial is over. They're not going to scale anymore,” Nicholson said.
To help end users scale their autonomous mobile robot (AMR) fleets, Slamcore’s spatial awareness systems can track the location of all vehicles in a warehouse - both manual and automated - which can enable fleet orchestration from a safety-first point of view.
“If we only care about the robots, then we are missing the big picture,” Nicholson said.
Deep learning, object recognition to improve throughput
Heterogeneous fleets of manual vehicles, autonomous vehicles, and people on the floor can allow warehouse operators to maximize throughput. But if robots lack the ability to understand what objects are around them to adjust their behaviors, engineering controls need to be implemented, which can actually hinder efficiency.
Engineering controls might include creating robot-only zones, or setting speed limits on autonomous vehicles. Nicholson said the current speed limits on robots are nowhere near the fastest speeds they can travel at, but restrictions are required for safety.
Automated guided vehicles (AGVs) use relatively simple localization systems, such as following a line or markers on the floor. Nicholson said although line following is a good localization technique, AGVs don’t have great perception abilities.
Object recognition allows computer systems to identify and label items within images. AGV operators can add Slamcore’s hardware box and cameras to their machine to add on the perception of a fully-autonomous robot, including both localization and object recognition.
“Vision really comes into its own, because you get so much more spatial data from a stream of images than you do from a bunch of laser measurements or from other ultrasonic measurements.”
Slamcore’s proprietary AI stack takes in images and outputs object positions with labels. Nicholson said deep learning and generative AI are making it easier for computers to identify objects.
“There's lots of companies out there who do labeling of images,” Nicholson said. “But if you don't know where that object is, you really limit the amount of value you can bring.”
Because Slamcore’s software is foundationally a localization system, the computer knows where the cameras are within the facility space, and therefore can tell robots both what things are in front of them and how far away they are. Based on that information, robots can modify their behaviors to operate safely.
If Slamcore’s software identifies a person, the robot might stop, slow down, or move out of the way. But if it detects a pallet, the robots don’t need to slow down, allowing them to operate at higher speeds without engineering controls.
“That's the real value of being able to identify the difference between objects in real time for the robot,” Nicholson said.
But Slamcore’s goal isn’t just to improve the capabilities of one robot. Position and object identity information can be shared across an entire fleet of vehicles in a warehouse. Fleet management and orchestration software can use real-time data to set up dynamic zones around obstructions with temporary speed restrictions.
Obstructions could be a stray pallet or box. But if it’s a person who entered an area they weren’t meant to be in, information can be relayed to both robots and human operators so they can know what to expect when they enter that area.
Dynamic zones have the potential not only to improve path planning by routing around obstructions - improving conveyance and picking efficiency - they can also bolster safety.
Object recognition can help increase precision
Slamcore’s algorithms analyze each frame of video before the next one is captured, quickly contextualizing the environment surrounding vehicles. That context includes localization, object detection, and object recognition.
Nicholson said object recognition can have a knock-on effect for positional accuracy. The whole point of SLAM is to create a map as vehicles move around an area. But measuring against other moving objects can create errors.
Lasers are great for capturing distance and angle measurements. But laser-based navigation systems can struggle in dynamic environments because they don’t have context to know if what they’re measuring against is stationary or in motion. That can make it hard to localize where vehicles are located within a facility.
Visual AI enables navigation systems to identify which object a measurement was taken from, whether it be a person, another vehicle, or a part of the building. Doing so allows navigation software to ignore static objects and focus on tracking dynamic objects.
Slamcore Aware for manual vehicles can provide positional accuracy within 20 centimeters, or within eight inches. Nicholson said customers who request accuracy within two centimeters - or within an inch - use additional inputs such as wheel odometry.
“I'm not a vision fundamentalist. I believe vision should always be present,” Nicholson said. “But it's not the only answer.”
He added accuracy within two centimeters can enable AMRs to work really well. Although that level of accuracy is possible with vision alone, fine-tuning and reliability become more difficult to maintain.
“We believe in, ‘Vision plus other sensors,’ if you want to go down to that level of accuracy,” Nicholson said. “Vision alone isn't the answer to full autonomy in this commercial, scalable way.”
That being said, spatial awareness systems like Slamcore can complement other sensors by reducing the precision necessary for those systems - and drive down costs.
Instead of relying on lasers that can measure close and far distances to localize machines precisely, Nicholson said vision-based SLAM running on cost-effective hardware can be paired with light lasers in the $100 range to achieve the same level of precision.
Developing visual SLAM required Slamcore to consider what the robots need to function, what facilities need to operate, and what the warehousing industry needs to improve safety and performance. Nicholson said lasers and lidar have been critical to the success of the industry, and SLAM machine vision is the thread that pulls everything together.
“If I was going to have a life-or-death decision on a sensor, I'd rather it was a laser than a probabilistic AI engine from a computer vision algorithm,” he added. “Or at least I want a few more years of testing in the field before I make that the goal.”
Generative AI can predict and prevent future accidents
The term digital twin can mean different things to different people. Nicholson said the value proposition of a perfect 3D reconstruction is not entirely clear to him, but a “spatial-temporal” digital twin can provide clear advantages.
“What I'm interested in is a digital twin which is much more a spatial-temporal representation of the location of every vehicle and every piece of material in real time,” he said. “It's really useful because you can do your real time orchestration, but also [look at] history.”
Nicholson described a warehouse fleet with machine vision equipped on every vehicle as a kind of “macro sensor,” with lots of cameras roaming around the warehouse that function as one big real-time data collection system.
Each camera knows where it is within the building, and together they are all constantly spatially indexing the whole facility, which can be used to map the location of inventory to form a database of information over time.
Slamcore’s spatial-temporal digital twin doesn’t require a database with petabytes of data because everything is logged in a simple format. Currently, that data includes an object name, a coordinate, and a timestamp. Because the data is numerical at its core, it’s easily searchable in real time.
Nicholson said generative AI is the perfect tool to extract meaningful information from the digital twin. Because all the information is interconnected through time and location, operators can use generative AI to ask questions about things like bottlenecks, pick time, and stocking strategies.
“You can start to add abstract questions to this because it'll have enough data to see over the last year across this site, ‘I know over the 1,000s sites I've been deployed on, I've seen these trends,’” he said.
With enough data, generative AI could also pull out trends about what sequence of events created future ones. Running in real time, it could start to predict seconds into the future, and maybe even a minute. “And then I think we're probably going to start to hit the limits of what's even possible,” Nicholson said.
Generative AI analysis could potentially predict accidents in real time if it sees a sequence of events that previously led to an accident. “That could massively reduce safety incidents, especially if you bring in near-miss data,” Nicholson said.
Predictive analysis would be difficult to sell as a standalone product. Doing so would require convincing customers to put hardware in the field with the promise of generative AI in the future.
But until generative AI can deliver that level of foresight, Nicholson said the machine vision cameras deployed by Slamcore are already providing useful localization and mapping data.
“Operations guys are saying, ‘Awesome. I want my entire fleet to have this, because this will actually help me right now,’” Nicholson said. “But it's laying the foundation for something much bigger.”
Want to learn more about machine vision? This article was featured in the August 2024 Robotics 24/7 Special Focus Issue titled “Machine vision to increase robot precision.”
Introducing Slamcore Aware at MODEX 2024
Slamcore CEO and co-founder Owen Nicholson describes Slamcore's news hardware and software package for manually-operated vehicles, which can offer real-time location tracking services.About the Author
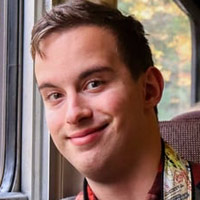
Follow Robotics 24/7 on Linkedin
Article topics
Email Sign Up
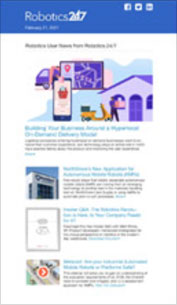
