Urbanization is Progressing at a Rapid Pace
The United Nations projects that by 2030 the number of so-called megacities of over 10 million inhabitants will rise to well above 40.
About two-thirds of the global population is expected to reside in cities by 2050.
Without a doubt, cities represent the epicenters of future global economic growth as well as the social, technological and cultural advancement of humanity.
The supply chain implications of these trends are profound. To remain competitive, companies across all sectors, industries, and markets need to succeed at serving urban customers and consumers.
This is particularly true in retail, where the battle over future consumer markets is largely a battle over who excels at managing the urban last mile of physical distribution.
Strategies for Change
As urban populations grow, cities are becoming an increasingly complex and uncertain operational environment.
The key driver of this complexity is a rapid increase in urban density. The potential of any city to grow in geographical size is naturally bounded.
At some point, cities find that to keep growing they must build upwards to accommodate more people, businesses, and activities.
However, these growth strategies also lead to rising levels of congestion across the urban transportation infrastructure and an increased likelihood of accidents and other random disruptions to urban mobility.
Planning efficient and reliable last-mile delivery operations is thus becoming more challenging and requires higher levels of operational flexibility and redundancy from urban distribution systems.
At the same time, the retail industry is facing a continuing boom in e-commerce together with rapidly rising customer expectations regarding the speed, timeliness, flexibility, and customization of last-mile delivery services.
Heavily cross-subsidized premium delivery services offered by some of the major e-commerce platforms are gradually educating consumers to further postpone their purchase decisions, as they can rely on the availability of affordable, fast-track delivery at all times.
This trend toward “on-demand consumerism” renders the last-mile performance of a company’s logistics operations a critical competitive factor.
However, it also leads to a massive increase in the spatial and temporal fragmentation of urban deliveries, making it increasingly difficult for logistics service providers to consolidate shipments into efficient delivery routes.
These trends could render the provision of delivery services to urban markets increasingly unsustainable unless companies fundamentally rethink their approach to last-mile logistics.
Without substantial paradigm shifts in the design, planning, and operation of last-mile logistics, urban goods distribution will become - and remain - economically unprofitable.
Moreover, delivery operations will burden urban environments with more traffic congestion and associated noise and emissions pollution.
One way in which companies can redefine last-mile logistics is by adopting anticipatory planning and flexible operations that allow for dynamic and pro-active adjustments to rapidly changing operational conditions and market requirements.
Another strategy is to build multi-echelon network architectures and multi-modal delivery models, integrating connected and potentially autonomous technologies into smart cyber-physical systems.
Both of these options require companies to deploy advanced analytics, powered by diverse sources of high-resolution data and supported by highly connected and sensor-equipped operations.
Mobile Data and Connectivity as Key Enablers
A number of companies, especially in the fast-moving consumer goods industry and in e-commerce, have realized that they need to challenge traditional last-mile delivery solutions in light of recent advances in data analytics and technology.
Supported by state-of-the-art research from MIT, some enterprises are leveraging their existing data sources. They are using transactional records, delivery data from individual routes, high-resolution telemetry and movement data on the level of individual vehicles to develop a more precise picture of their last-mile logistics operations.
Similarly, publicly available data sources are becoming increasingly relevant for the optimal design and planning of last-mile delivery systems and processes.
These data sources provide insights into the operational and commercial environment - from road infrastructure characteristics to traffic and congestion dynamics to sociodemographic profiles of the customer base.
These are important advances - but they have certain limitations. Most of these data sources describe urban markets in retrospect; many are even static in nature.
This information is valuable in discovering and understanding systematic patterns in how urban markets and the associated logistics services function and perform historically, and in predicting how they may evolve in the future.
However, the information is limited in its potential to improve the way companies dynamically react and proactively adapt to short-term fluctuations in the marketplace and the operational environment on tactical and operational levels.
With the rise of the Internet of Things (IoT) sensor networks, companies are now able to obtain reliable, high-resolution and real-time visibility into their transportation networks.
Moreover, this visibility is not limited to the status and location of shipments, or to the inventory levels of individual storage locations. It is now possible to obtain system-wide insights into the number, status, location and expected trajectory of products and shipments along their entire journey from their upstream source or vendor to the final recipient.
Driven by significant advances in sensor technology, mobile computing, mobile data and vehicle connectivity, real-time visibility is no longer limited to aggregate information on stationary items at distribution and warehousing facilities.
It is now possible to achieve real-time visibility for in-transit volumes down to the level of individual items or shipments. This new level of detail and dynamism enables companies to substantially reduce the number of blind spots in their distribution networks.
Previously, these blind spots prevented them from dynamically adjusting their last-mile distribution approach to changing market dynamics, or to proactively mitigate the effects of disruptions to their last-mile operations.
The Data Analytics Revolution
Another key development is the availability of advanced analytics. The recent methodological advances in this field will have a major impact on last-mile logistics on three levels.
First, advanced analytics - and in particular a group of tools commonly known as machine learning - can help companies to better understand urban markets, customers, and operational environments.
This is particularly relevant when serving a large and highly fragmented customer base.
Such tools can provide substantial business value by revealing customer-specific insights that would have otherwise remained hidden or too costly to identify.
For instance, a leading global brewing company recently partnered with researchers from the MIT Megacity Logistics Lab to use data to improve the operational efficiency of its global last-mile delivery operations.
Based on historic route plans and delivery records, machine learning tools helped identify customer-specific delivery constraints in a pool of hundreds of thousands of customers around the world - from big box retailers in the U.S. to single-owner operated nano stores in emerging markets.
The analyses helped to identify those customers that are most disruptive to the efficient operation of that company’s delivery operations due to their hidden delivery constraints.
The company was then able to address these issues by re-incorporating the information into its route planning algorithms, or by reconfiguring distribution services for certain customers.
Similarly, machine learning can help reveal and exploit the local knowledge and expertise of a company’s distribution workforce.
For instance, the analysis of high-resolution GPS traces in conjunction with telemetry data and transactional records can provide relevant insights on the availability and suitability of local infrastructures such as roads and parking bays for last-mile delivery.
The data can reveal behavioral patterns of drivers and delivery crews that have local knowledge about their route territory and know better than any algorithm or data source where to park, which shortcut to take, or which congestion hotspot to avoid.
Extracting this knowledge without having to disrupt crew member workflows can achieve significant improvements in route planning and more effective delivery instructions.
Companies also can maximize service levels and minimize cost inefficiencies due to inaccurate planning.
Second, state-of-the-art analytics of the increasing amounts of readily available, high-resolution data can help predict last-mile operations at much higher levels of detail and accuracy.
In light of rapidly increasing customer expectations regarding delivery speed and responsiveness, detailed and accurate spatiotemporal demand forecasts - i.e. understanding what, where and when customers will order - are becoming indispensable.
At the same time, shorter delivery lead times give companies limited leeway to compensate for short-term fluctuations in traffic conditions or the availability and pricing of (potentially crowd-sourced) carrier capacities.
Advanced analytics can also be used to develop reliable near-term predictions of traffic dynamics and resource availabilities, taking into account historic patterns as well as real-time information on influencing factors such as the weather or other relevant events.
Such capabilities are crucially important to guaranteeing high levels of service to increasingly demanding customers.
Lastly, advanced analytics can inform a new generation of prescriptive analyses, helping companies make the right tradeoffs and decisions during the strategic design, tactical planning and day-to-day operations of fast, reliable and responsive last-mile distribution systems.
More companies across sectors, industries, and geographies are reaching out to academic partners to develop prescriptive decision support tools based on high-performance optimization and simulation techniques.
Questions and Answers
To gain an understanding of how analytics can be deployed to improve last-mile logistics, it’s helpful to look at specific questions that the technology can address.
Changing urban environments and customer needs require new approaches to the design of urban distribution networks.
They also raise several questions for supply chain managers and logisticians.
For instance, how can a company transition from a traditional, static, single-tiered, uni-modal urban distribution approach that is built around various dimensions of operational flexibility?
Especially when such a network must also allow for dynamic changes in the company’s distribution approach in response to dynamically unfolding customer requirements and operational conditions such as traffic or weather.
And, how can enterprises design, plan and operate a distribution network that is built on a combination of various vehicle technologies, delivery models and supporting distribution facilities?
Large-scale optimization models informed by descriptive and predictive analyses of both historic and real-time data can provide answers to these and other questions.
Faster, more responsive last-mile delivery services can also have major implications for inventory management - raising more questions.
How, where and in what quantities should a company allocate its inventory so that it can quickly respond to customer requests at various service levels?
What is the impact of decentralizing inventory in a network of hyper-local storage locations in order to move closer to the urban customer?
Can service levels be improved and cost reduced by sharing inventories across a network of fulfillment locations, or by making them mobile?
Simulation and analytical approximation methods can provide insights to these questions, based on an in-depth understanding of the spatiotemporal dynamics of urban demand obtained from advanced analytics of last-mile Big Data.
At the operational level, more flexible delivery models require more sophisticated route planning capabilities.
Logisticians must ask: How should delivery vehicles be dynamically routed in response to unfolding uncertainty of demand and operational conditions?
How can multi-tiered and multi-modal delivery models that rely on multiple vehicle systems that work hand-in-hand be perfectly synchronized into an efficient and reliable service?
How will the introduction of (partially) autonomous technology and unmanned aerial or terrestrial vehicles affect the design, planning, and operation of such systems?
These and other questions can only be answered by smartly combining large-scale optimization, high-resolution simulation, and detailed near-term prediction of market dynamics, environmental conditions and delivery operations.
Communicating About Data
In an increasingly customer-centric and service-oriented market environment, companies need to take a leap of faith to embrace these new methods of leveraging data and analytics for their strategic, tactical and operational decision making.
To many companies, this appears particularly challenging because the use of data and analytics is not ingrained in their corporate culture.
What’s more, many lack the internal capabilities to develop such tools on their own.
To avoid being blindsided by the analytics revolution in last-mile logistics, many companies need to build internal capabilities and collaborate with external knowledge centers such as academic institutions.
Moreover, they need to initiate a cultural change that enables them to develop and apply advanced analytics and quantitative methods both tactically and strategically.
For example, some companies - even in traditional industries - are turning advanced analytical methods from black boxes into trusted toolsets by building advanced analytics teams and centers of excellence that operate outside of their day-to-day business operations.
These teams identify issues that benefit the most from analytical approaches to problem-solving and provide the expertise that business units and, ultimately, the whole organization, need to put these solutions into practice.
Turning advanced analytics into actionable insights, however, requires a second group of people that is often neglected in companies’ efforts to build analytics expertise - the data translators.
Data translators bridge the gap between advanced analytics experts (usually referred to as data scientists) and domain experts who run the business on a daily basis.
Educational institutions such as MIT should develop graduate and professional programs that educate supply chain and logistics professionals to become data translators.
The industry needs to engage with academia to align research agendas and academic curricula with the real-world challenges faced by practitioners in making the next generation of last-mile distribution systems become a reality.
Visualization has a key role to play in achieving these goals.
Current display standards, as well as rapidly emerging technologies such as augmented and virtual reality, allow for rich, high-fidelity visual representations of complex datasets along with the mechanics and results of advanced analytical methods that are built on top of these datasets.
These visual representations are a powerful tool that enables domain experts to understand, validate and trust the data and the outcomes of sophisticated analyses.
Individuals can interact with these representations and conduct what-if analyses, or move from high-level, aggregate views of supply chains to arbitrarily granular views.
As technology evolves, interactive visualization will become an indispensable decision-making tool.
To support the logistics industry in embracing this development, the MIT Center for Transportation and Logistics has partnered with leading companies to conduct research and develop tools at the intersection of last-mile logistics, advanced data science, and interactive information visualization within the newly created Computational and Visual Education (CAVE) Lab.
Benefits from Sharing Data on a System Level
Aside from promoting the use of data and analytics within individual companies, a huge untapped potential to improve the efficiency and long-term sustainability of urban last-mile delivery operation lies in cross-vendor collaboration and data sharing.
Today’s last-mile operations are highly fragmented across multiple carriers and logistics service providers, leading to suboptimal asset utilization.
Hundreds of thousands of unnecessary miles and hours traveled are expended within already congested and polluted urban centers.
There is fierce competition for the increasingly scarce resource of urban transport infrastructure capacity.
Attempts by policymakers and legislators around the world to force vendors to collaborate, coordinate and consolidate urban shipments have largely failed.
The solution to the problem may, once again, be technology. Technological advances are enabling increasingly seamless, low-cost and platform-independent connectivity between vehicles, distribution facilities, infrastructure components, shippers, carriers, and customers.
The transaction cost, risk, and operational friction caused by sharing information and assets across players will reduce substantially over the coming years.
This trend may be reinforced by technologies such as blockchain that reduce the effort, risk and cost of validating information, executing contractual agreements and processing transactions of money, goods, and services further.
With regards to system-level connectivity and data sharing, there are four major directions in which urban last-mile delivery operations will experience transformational shifts driven by new levels of connectivity in the near future:
- Vendor-to-vendor, carrier-to-carrier, and vendor-to-carrier connectivity will finally enable the safe, efficient and mutually beneficial sharing of transportation infrastructure, facilities and fleets; a tight coordination and consolidation of shipments across vendors and carriers; and an efficient integration of downstream last-mile delivery operations, upstream first-mile pick-up operations, and reverse logistics flows.
- Vehicle-to-infrastructure connectivity will allow for a paradigm shift away from individual-vehicle route optimization toward a system-level optimization of overall urban mobility by jointly optimizing vehicle flows and sharing information about traffic, accidents, the availability of parking spaces and other relevant operational constraints to last-mile delivery.
- Machine-to-machine connectivity will enable more streamlined and efficient interactions between multiple vehicle systems (e.g., trucks and cargo cycles or bike couriers) in multi-modal delivery models. It is also a crucial prerequisite for the large-scale deployment of autonomous vehicle systems for last-mile delivery.
- Carrier-to-cloud or crowd-to-cloud connectivity will encourage the massive sharing of (potentially crowdsourced) historic and real-time information on road and traffic conditions, customer-specific delivery requirements and other defining actors of last-mile efficiency.
The latter trend will also enable new commercialization models for last-mile data and analytics as a service.
Already, some of the major express logistics and parcel delivery services are investing heavily in the analysis and commercialization of data that their vehicle and courier fleets can collect in cities around the world, every day of the year.
Future Challenges
Rising demand from consumers, who are increasingly intolerant of service failures, coupled with urbanization and more intense competition is forcing companies to rethink their last-mile delivery supply chains.
Developments in data analytics will play a key role in meeting these challenges. But first, companies must build capabilities that allow them to use analytics creatively, and, crucially, to have the confidence to implement solutions based on these analyses.
About the Author
Matthias Winkenbach, Ph.D., is director of the MIT Megacity Logistics Lab. He can be reached at [email protected].
The End of Distribution As We Know It?
In an uncertain future, one thing’s for sure: The era of the vast, single-use, single storey, plain-vanilla warehouse is over
Warehouses of the future with on-demand additive manufacturing capabilities are co-located in multi-modal centers for efficient transfer of goods.
While businesses of all sizes and industries are scrambling to keep up, distribution is being upended entirely.
Some experts have suggested that in 30 years, the distribution industry as we know it today will disappear altogether. But that doesn’t mean that demand for warehouse space is going to go away.
In fact, demand is rising. Major population hubs are driving a marked increase in industrial absorption. The Journal of Commerce reports that as of first quarter 2017, US vacancy rates for warehouse and distribution space were at a 17-year low, with asking rents rising quarter over quarter since 2015.
“The proliferation of online retail is creating structural shifts in the industrial sector through a reconsideration of retail space needs and supply chains”
Retailers are increasingly taking advantage of major savings by maintaining inventory off-site, even as they absorb vacant or underdeveloped properties in densely populated urban areas so they can store goods closer to their end users - and get them delivered faster.
Download the Paper Roadmap for Change: The Flexible Industrial Distribution Facilities Network of the Future
Article topics
Email Sign Up
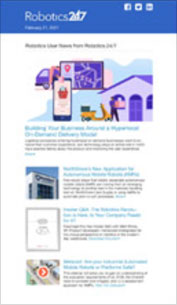
