The Motion Research Group at the University of Waterloo’s Centre for Automotive Research (WatCAR) has been contributing to the body of research around autonomous vehicles by working on a project related to self-driving vehicles, led by Professors Krzysztof Czarnecki and Steven Waslander.
The project, known as Autonomoose, is an autonomous drive-by-wire vehicle project that operates within WatCAR. Its objectives include improved self-driving in extreme weather conditions and during emergency maneuvers to avoid obstacles.
“Our team has many researchers working on autonomous cars and we wanted a real-world vehicle platform on which to test new research theories and algorithms,” Czarnecki says. “We are working on the future of automotive transport, and we now have a state-of-the-art vehicle platform on which to test the real-world performance of our theories and controllers.”
Czarnecki and Waslander have been working on the project for the past two years and oversee the planning and navigation research for the project. They recently invited John McPhee, a Canada research chair and professor of Systems Design Engineering at the University of Waterloo, to improve the motion controller for the vehicle and to oversee the dynamics and control aspects of the project. The research team selected a 2015 Lincoln MKZ Hybrid as the vehicle platform, and used Maplesoft’s Maple and MapleSim to support their research. They outfitted the car with the sensors and actuators required for implementation as an autonomous vehicle.
“The Lincoln MKZ is the vehicle preferred by AutonomouStuff, the company that converted the MKZ to a drive-by-wire vehicle,” McPhee says. “We could then add our own sensors—Lidar, cameras, etc.—and control systems to convert the vehicle to be fully autonomous.”
The vehicle is controlled by actuating the accelerator pedal, brake pedal and steering wheel to track a reference trajectory provided by a higher-level decision making architecture. To design effective and robust trajectory tracking controllers for the vehicle, the true vehicle dynamics must be modelled. The two models that must be constructed and identified are of the powertrain and of the vehicle’s longitudinal and lateral dynamics.
“High-fidelity vehicle models allow us to design controllers based on model-in-the-loop simulations of vehicle performance,” McPhee says. “In addition, they inform the design of low-fidelity models that we will implement in model-predictive trajectory tracking controllers.”
Incorporating the Maplesoft Platform
The team chose Maplesoft’s modeling and simulation tool MapleSim, supported by the symbolic computation tool Maple. MapleSim offers a comprehensive simulation platform to develop and test the vehicle models, supported by Maple, the computational speed of which aids the team in carrying out the required calculations quickly. The team needed fast simulations and model-based controllers to achieve real-time control of vehicle motion, and for-testing sensors. The team also needed a powerful simulation environment to analyze navigation algorithms.
Using MapleSim, the team created high-fidelity MapleSim models of the Autonomoose that were validated using four-post testing, rolling dyno testing, and track testing on the Waterloo test track and the Waterloo airport runways. Optimized simulation code was then exported from MapleSim and used to simulate the vehicle motion in virtual environments created using Unreal Engine 4 and other VR software.
The goal of the powertrain model was to obtain a mapping of control inputs (accelerator and brake pedal positions) and vehicle states (wheel speeds, vehicle speed, etc.) to the torque applied at the wheels. A high-fidelity model of the Lincoln MKZ`s hybrid powertrain included the architecture of the vehicle`s powertrain control module as well as the dynamics of the drivetrain system.
To identify such a model, the team needed to obtain measurements of many CAN bus signals, including demand torques, observed torques, and speeds of the engines and electric motors. CAN is a vehicle bus standard designed to allow microcontrollers and devices to communicate with each other in applications without a host computer.
Goal of the Model
The goal of the vehicle dynamics model was to determine how the vehicle will respond to steering wheel inputs as well as torque transmitted to the wheels from the driveline. A high fidelity model of the Lincoln MKZ’s vehicle dynamics considered its yaw, pitch, and roll inertias as well the suspension's kinematic and dynamic parameters. “Many of these parameters can be determined from measurements using our own equipment, but the semi-active suspension of the Lincoln MKZ adds additional complexity to the model,” McPhee says.
To achieve real-time control of vehicle motion, the team used MapleSim’s model reduction features to create simpler control-oriented models of the Autonomoose that are embedded in model-predictive controllers. Essentially, these models predict the vehicle response to inputs over some horizon, which is used to optimize the inputs over the next timestep, McPhee says.
“Symbolic computing was used to obtain exact gradients, Lagrangians, and Hessians that speed up the convergence rate of our optimization methods,” he adds. “Finally, the model-predictive controllers were tested on the high-fidelity model to make sure that the controllers would perform safely and optimally on the actual vehicle.”
The team has achieved longitudinal control and is now working on solving lateral control issues so the vehicle can safely avoid other vehicles and moving obstacles on the roads, while navigating its designated route.
“This requires a very good understanding of vehicle and tire dynamics, which we’ve been able to achieve through our MapleSim model and vehicle testing,” McPhee says. “In recent track testing with our longitudinal controller, we were able to significantly increase the operational speed of the Autonomoose. In fact, we were able to get it up to the limit allowed by our test track. This was a major achievement by our research team.”
The team also wants to achieve a moving-horizon estimation (MHE) of unknown vehicle states and parameters, such as road friction.
“It's impossible to control a dynamics system without knowing its current states, so the team uses a model combined with measurements in order to estimate the unknown states,” McPhee says. “MHE is highly-complementary to our model-predictive controllers, and we are exploiting the same symbolic computing features to develop new moving horizon estimators.”
Some of the ideas and data resulting from the project are being used in other machine learning research projects in McPhee’s group, such as optimal control of exoskeletons and stroke rehabilitation robots. Like the Autonomoose, these are assistive devices that require high-performing model-based controllers that run in real-time due to the advantages of tools like MapleSim and Maple.
Sources: Press materials received from the company and additional information gleaned from the company’s website.
About the Author
Press releases may be sent to them via [email protected]. Follow Robotics 24/7 on Facebook
Follow Robotics 24/7 on Linkedin
Article topics
Email Sign Up
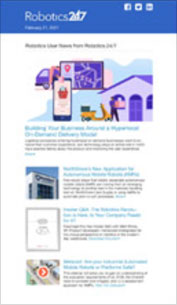
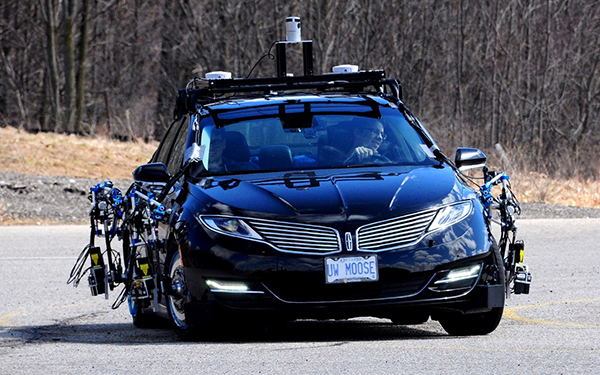