For robots, drones, and autonomous vehicles to become more agile in dynamic environments or around people, they need to learn to see more clearly. The Toyota Research Institute today announced that the International Conference on Computer Vision has accepted six research papers on machine learning. The research advances understanding across various tasks crucial for robotic perception, including semantic segmentation, 3D object detection, and multi-object tracking, said Toyota.
Over the past six years, the Toyota Research Intitute (TRI) said its researchers have made significant strides in robotics, automated driving, and materials science in large part due to machine learning—the application of computer algorithms that constantly improve with experience and data.
“Machine learning is the foundation of our research,” stated Dr. Gill Pratt, CEO of TRI. “We are working to create scientific breakthroughs in the discipline of machine learning itself and then apply those breakthroughs to accelerate discoveries in robotics, automated driving, and battery testing and development.”
Simulation leads to 'scalable supervision'
As the International Conference on Computer Vision (ICCV) began this week, TRI shared six papers demonstrating its research in machine learning, including geometric deep learning for 3D vision, self-supervised learning, and simulation-to-real or “sim-to-real” transfer.
“Within the field of machine learning, scalable supervision is our focus,” said Adrien Gaidon, head of TRI’s Machine Learning team. “It is impossible to manually label everything you need at Toyota's scale, yet this is the state-of-the-art approach, especially for deep learning and computer vision.”
“Thankfully, we can leverage Toyota's domain expertise in vehicles, robots, or batteries to invent alternative forms of scalable supervision, whether via simulation or self-supervised learning from raw data,” Gaidon said. “This approach can boost performance in a wide array of tasks important for automated cars to be safer everywhere anytime, robots to learn faster, and battery development to speed up lengthy testing cycles.”
In the six papers accepted at ICCV, the researchers reported several key findings. Notably, they show that geometric self-supervised learning significantly improves sim-to-real transfer for scene understanding.
The resulting unsupervised domain adaptation algorithm enables recognizing real-world categories without requiring any expensive manual real-world labels, said TRI.
Synthetic data can lead to greater cognitive ability
In addition, TRI's research on multi-object tracking found that synthetic data could provide machines with cognitive abilities like object permanence that have been difficult for machine learning models but are easy for most humans. This new development increases the robustness of computer vision algorithms, making them more aligned with people’s visual common sense, said Toyota.
TRI also said its research on pseudo-lidar shows that large-scale, self-supervised pre-training can boost the performance of image-based 3D object detectors. The proposed geometric pre-training enables training powerful 3D deep-learning models from limited 3D labels, which are expensive or sometimes impossible to get from images alone.
More information about all six papers and TRI’s machine learning work can be found on its Medium page or at TRI's presentations at ICCV.
Article topics
Email Sign Up
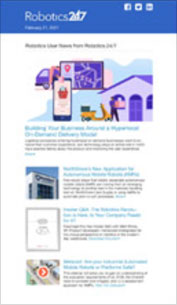
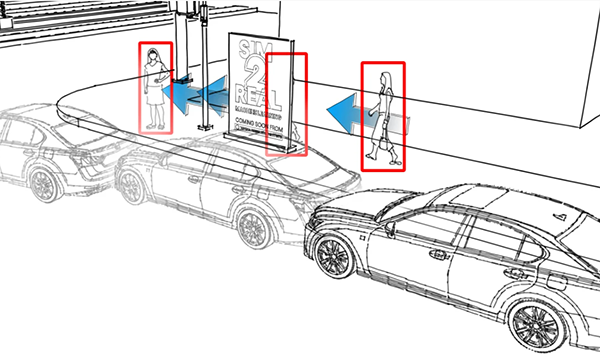