Technologically, the last 30 years or so have been shaped by advancements in computation, and the ability to build machines that can make decisions independent of human operators is a direct result of this progress.
With the growing global demand for machines that can perform labor, intelligent automation will bring about the real changes needed to deliver at scale.
Though historically, robotic systems with embedded intelligence are inherently more difficult to build with reliability because they operate in the real world — a world with less regularity and more unpredictable consequences than the carefully-designed frameworks of the digital world.
Given the challenges, it is not surprising to consider that factory automation is still largely driven by human workers who perform tasks that are often repetitive, but difficult to automate.
Automation is non-trivial, but it is not due to the fact that research cannot solve a lot of these problems — but that it only became a possibility more recently. There are certainly quite a few reasons for this, some of which have to do with the hardware and computational advancements, and others that revolve around data. But there is another interesting theory going around that is articulated by Sara Hooker in “The Hardware Lottery”.
She postulated that research directions in the field of machine learning are often explored due to software and hardware available at the time, rather than being motivated by the most promising ideas. This theory is aligned with our premise at RIOS that advancing the capabilities of robots is heavily dependent on both specialized hardware and software that must coevolve.
Today, we are reaching an inflection point, and there is a monumental opportunity to develop custom hardware and software systems that enable robots to take on increasingly open-ended tasks without the need of reprogramming for each new instruction.
We can do this by taking the lessons of the internet to apply data at scale to robotics. By strategically designing systems with the intent of learning from them, and building the infrastructure to support information sharing, we can adapt more quickly to new tasks and master the ones we are already familiar with.
The real promise of applying machine learning to robotics is not teaching a single robot to learn for itself, but to aggregate experience from a vast network of robots so that they can improve at scale.
A core tenet of what we do at RIOS is to build machines with this idea in mind. Like hardware, skills and behaviors should be transferable across platforms when possible, and each deployed system should be able to share what it has learned with other systems. At a high level, you can think of this as storing knowledge rather than just data to reduce the need for retraining.
The result is a class of robots that can do a variety of tasks and address new challenges with less development time. By building distributed robots that continuously learn from both their environment and the collective experience of others, we can help push intelligent robotics forward at scale much in the same way that the information economy benefited from the web.
The next generation of technological progress is starting to favor organizations that can rapidly assemble the best technologies of the web-era and use them to take fields like robotics in new directions. In many parts of the world, where labor shortages exist or workers are subjected to poor conditions, this couldn’t come at a better time.
Moreover, what used to be a long lead-time in deploying new systems or developing solutions is disappearing as robots can reuse not just hardware, but prior knowledge when taking on new tasks. As more robots fill empty roles in factories, we’ll start wonder how we lived without them, and eventually forget they are doing the most thankless of work for us without any complaints.
Matt Shaffer
is the RIOS Director of Artificial Intelligence and is the architect behind the brain of our robots. This article is a shortened version of Matt’s article Scaling Artificial Intelligence for Robotics in 2021.
Article topics
Email Sign Up
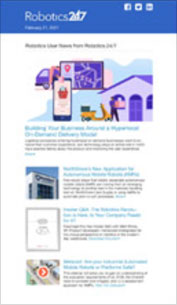