Robot Era, a Beijing-based humanoid robot startup, announced that it will open-source its reinforcement learning (RL) framework Humanoid-Gym on GitHub.
The move is intended to lower the threshold of developing RL algorithms for humanoid roboticists worldwide, providing them with a practical platform to build on to avoid “reinventing the wheels.”
Reinforcement learning options on Robot Era robots
Robot Era, according to the company, is one of the first players in the industry to showcase a humanoid robot's locomotion ability based on end-to-end reinforcement learning.
Founded in August 2023 and incubated by Tsinghua University's Institute for Interdisciplinary Information Sciences, Robot Era works to develop embodied AI, humanoid robotic technologies and products.
Due to the complex structure and control mechanisms, humanoid robots pose a bigger challenge than quadrupedal robotics in the transition from a simulation avatar to a working prototype in the real world.
In the video above, the company's full-size humanoid robot, XBot-L, can be seen walking on slippery snow and climbing up and down stairs with stability and dexterity. The movements serve as part of a test on the robot's locomotion capabilities.
This process is known as Sim-to-real policy transfer in the parlance of reinforcement learning.
Based on the specific reward functions and domain randomization signals, the Humanoid-Gym framework from Robot Era can help simplify the steps humanoid robots need to take to achieve Sim-to-real translation, and according to the company, streamline the development process to reduce technical difficulty and bolster engineering productivity.
The framework was previously deployed and successfully tested on Robot Era's two models, XBot-S and XBot-L.
Another benefit of Humanoid-Gym, according to Robot Era, is that it is calibrated to allow for proof-of-concept tests on humanoid robots in MuJoCo, an advanced simulator for multi-body dynamics. This calibration allows for close alignment between a simulated and real robot model.
The framework also introduced several variables to assess the reinforcement learning results, including speed tracking and the smoothness of robots.
Article topics
Email Sign Up
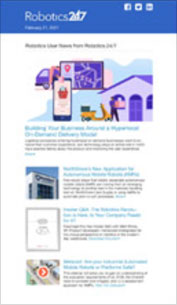
