Artificial Intelligence in the Supply Chain
There’s a lot of buzz and hype about artificial intelligence (AI) in supply chain management (SCM).
That’s understandable given its potential.
AI can offer a huge benefit to supply chain managers, but only if it is based on solid fundamentals that take into account the diverse and dynamic nature of today’s modern supply chains.
More importantly, it needs to consider the availability of the timely and accurate data needed to make smart decisions.
Before addressing what AI can do, it is critical to first understand what it is. In the simplest terms, AI is intelligence exhibited by machines, or when machines mimic or can replace intelligent human behavior, such as problem-solving or learning.
In essence, AI are machines making decisions whether that is deciding which chess piece to move where, or how to adjust an order forecast based on changing demand.
Despite its benefits, when looked at through the lens of a supply chain executive, AI is relatively useless unless it’s able to add value to support better decision-making.
Why AI Hasn’t Delivered in Supply Chain Management
In the race to use AI, many companies have made attempts to implement it, but the results have been disappointing. This is because of typical SCM systems today:
- Require armies of expensive planners
- Run complex engines at each step in the process and at each node in the supply network
- Are usually in conflict with other functions and/or partners
- Miss huge opportunities hidden in the network because they are locally sub-optimized
- Work on stale data and thus promote bad decisions
- Use dumbed-down, over-simplified problem models that do not relate to the real world
These SCM limitations have severely suppressed return on AI investments. For example, typical Retail/CPG supply chains still carry 60-75 days of inventory. The average service level in the store is about 96 percent, with promoted item service levels much lower in the 80 percent range.
The Casual Dining segment, on the other hand, carries around 12 – 15 days of inventory with relatively high waste and high cost-of-goods-sold. So, unless AI can make a significant impact on these metrics, it’s simply not delivering.
Key Requirements for AI in Supply Chain Management
Having worked with hundreds of supply chain executives, on dozens of software implementations, I’ve studied the AI issue a lot.
What I have found is there are eight criteria that are required for a successful AI implementation. Miss one of these and you’ll be lucky to achieve mediocre outcomes, but when you meet them all, you can indeed achieve world-class results.
For the AI solution to offer optimal value in the supply chain, it important to ensure the following fundamentals:
1. Access to Real-Time Data
To improve on traditional enterprise systems with older batch planning systems, new AI systems must eliminate the stale data problem. Most supply chains today attempt to execute plans using data that is days old, but this results in poor decision-making that sub-optimizes the supply chain or requires manual user intervention to address. Without real-time information, an AI tool is just making bad decisions faster.
2. Access to Community (Multi-Party) Data
The ability to access data outside of the enterprise or, more importantly, receive permission to see the data that is relevant to your trading community, must be made available to any type of AI, Deep Learning or Machine Learning algorithms.
Unless the AI tool can see the forward-most demand and downstream supply, and all relevant constraints and capacities in the supply chain, the results will be no better than that of a traditional planning system. Unfortunately, this lack of visibility and access to real-time, community data is the norm in over 99 percent of all supply chains. Needless to say, this must change for an AI tool to be successful.
3. Support for Network-Wide Objective Functions
The objective function, or primary goal, of the AI engine must be consumer service level at the lowest possible cost. This is because the end-consumer is the only consumer of true finished goods products. If we ignore this fact, trading partners will not get the full value that comes from optimizing service levels and cost to serve, which is obviously important as increased consumer sell-through drives value for everyone.
Further enrichment of the decision algorithm should support enterprise-level cross-customer allocation to address product scarcity issues and individual enterprise business policies. Thus, AI solutions must support global consumer-driven objectives even when faced with constraints within the supply chain.
4. Decision Process Must Be Incremental and Consider the Cost of Change
Re-planning and changing execution plans across a networked community in real-time can create nervousness in the community. Constant change without weighing the cost of the change creates more costs than savings and reduces the ability to effectively execute. An AI tool must consider trade-offs in terms of cost of change against incremental benefits when making decisions.
5. Decision Process Must Be Continuous, Self-Learning and Self-Monitoring
Data in a multi-party, real-time network is always changing. Variability and latency is a recurring problem, and execution efficiency varies constantly. The AI system must be looking at the problem continuously, not just periodically, and should learn as it goes on how to best set its own policies to fine-tune its abilities. Part of the learning process is to measure the effectiveness “analytics,” then apply what it has learned.
6. AI Engines Must Be Autonomous Decision-Making Engines
Significant value can only be achieved if the algorithm can not only make intelligent decisions but can also execute them. Furthermore, they need to execute not just within the enterprise but where appropriate, across trading partners. This requires your AI system and the underlying execution system to support multi-party execution workflows.
7. AI Engines Must Be Highly Scalable
For the supply chain to be optimized across an entire networked community of consumers to suppliers, the system must be able to process huge volumes of data very quickly. Large community supply chains can have millions if not hundreds of millions of stocking locations. AI solutions must be able to make smart decisions, fast, and on a massive scale.
8. Must-Have a Way for Users to Engage with the System
AI should not operate in a “black box.” The UI must give users visibility to decision criteria, propagation impact, and enable them to understand issues that the AI system cannot solve. The users, regardless of type, must to be able to monitor and provide additional input to override AI decisions when necessary. However, the AI system must drive the system itself and only engage the user on an exception basis, or allow the user to add new information the AI may not know at the request of the user.

AI in the Real World Today
Sounds good in theory, but how does it work out in practice?
Now that we have addressed the key fundamentals, let’s look at how some actual companies have achieved applying these criteria.
For instance, one of the major problems in Casual Dining is anticipating and meeting the demand for the restaurants, corporate-owned or franchised.
This is especially important during Limited Time Offers (LTOs).
Read: A New Approach to Digitial Transformation of the Restaurant Supply Chain
Using the eight criteria outlined above, a global, casual dining company connected to a real-time, multi-party network, and was able to rapidly achieve their objective function - excellent customer service at the lowest cost.
The company constantly monitors Point-of-Sale (POS) data and is using AI agents to recognize and predict the consumption patterns of consumers. In addition, intelligent AI agents create the demand forecast and then compare it to the actual demand in real-time.
When there is a significant deviation, the agents make the decision to adjust the forecast, and additional agents adjust replenishments. They then propagate those adjustments across the supply chain to trading partners in real-time at all times considering the cost of change and the propagation impact.
This drove a remarkable improvement in forecast accuracy. During promotions, the company achieved over 85 percent forecast accuracy at the store level and even higher at the DC level. This represents at least a 25 percent improvement over traditional approaches.
Intelligent agents also optimize restaurant orders autonomously by recognizing the impact of projected restaurant traffic trends and impact on LTOs and therefore the orders. The system runs on an exception basis but allows the managers to review the decision criteria and override orders where the managers may have local information such as inventory issues or local store traffic issues.
This has resulted in much faster order placement and order accuracy of over 82 percent, which reduces both inventory and waste dramatically while increasing service levels to the consumer. This is a significant improvement to all other known implementations in the marketplace.
Because the algorithms are highly scalable, they are processing over 15 million stocking locations continuously throughout the day.
Prior to the AI-based, multi-party execution system, restaurant managers had to interact with nine different ordering systems and manually create their own orders based on general guidelines, rules of thumb, and spreadsheet-based or manual calculations.
With AI implemented on a sound foundation, this company can now anticipate, manage, and serve demand at the lowest possible cost. During LTO’s, when demand fluctuations would overwhelm a restaurant manager, intelligent agents monitor demand in real-time, and autonomously orchestrate the supply chain to align supply with demand. Thus, the company can meet its goal and maintain high service levels while reducing the cost to serve.
These are not isolated results. Also in the food marketplace, another CPG-Retail implementation achieved 99 percent in-stock, in-store, with 25 days of supply (DOS) across the supply chain.
The inventory results are less than half the standard DOS in this marketplace and 3 percent points higher in in-store in-stocks AI-based solutions are being deployed at two large automotive tiers one suppliers with results ranging from 16 – 40 percent reductions in inventory as well as significant reductions in expedited freight costs.
AI Delivers Value in SCM Today
As you can see, laying the proper groundwork for AI pays huge dividends. There’s no doubt that AI offers even greater promise in the future, but, as these results show, there are significant benefits and dramatic results waiting for companies that focus on the fundamentals and put AI to use today.
The beauty of AI-based solutions is that they learn and drive continuous improvement over time. They get more precise and sophisticated as they gather more data and more experience.
The sooner you start, the better the results you’ll see in future, and the further ahead you will be.
With the right AI solution in place, you can outpace your competitors today, and be well-positioned for reaping even bigger rewards of AI’s promise tomorrow.
About the Author
Greg Brady is CEO and founder of One Network Enterprises. Brady founded the company in 2002 and is a renowned supply chain and technology visionary. Before that, he led i2 Technologies to high growth and market leadership for seven years, both as CEO and head of worldwide operations. Previously, Brady was vice president of worldwide applications marketing for Oracle and held positions at J.D. Edwards and at McCormack and Dodge. He holds a Bachelor of Science from Indiana University.
Related White Papers
8 Keys to Achieving Success with Artificial Intelligence in Supply Chain
This white paper looks at the fundamentals that supply chains need in place in order to achieve real results from Artificial Intelligence implementations. Download Now!
Can Blockchain Revolutionize the Supply Chain?
In this white paper Ranjit Notani, One Network CTO examines Blockchain’s powerful potential as well as a major problem and whether and how Blockchains can revolutionize the Supply Chain. Download Now!
The Internet of Things and Your Supply Chain
This white paper offers a quick and clear guide to the Internet of Things (IoT), it explains why this technology is so exciting for supply chain and offers a few examples of how it and related technology can be used to dramatically cut costs. Download Now!
Article topics
Email Sign Up
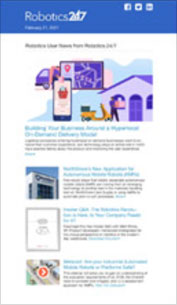
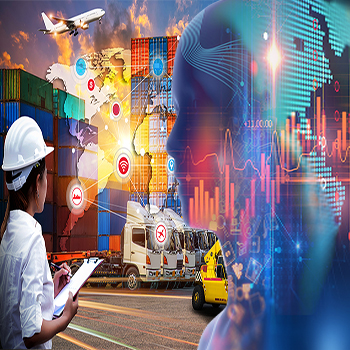